Information Visualization
[gL.edu] This article gathers contributions by Lara dos Santos, Johannes Richter, developed within the context of the Conceptual clarification about "Information, Knowledge and Philosophy", under the supervisión of J.M. Díaz Nafría.
Summary
In this article the field of information visualization is introduced. Beginning with a discussion on the difference between information and data, various theories on the meaning of information are being mentioned. The article continues to illuminate the meaning of knowledge and its correlation to data, followed by an introduction to the field of visualization. In this context, the four-sides model of the German psychologist Schulz von Thun is being introduced and brought into relation with the subject of information visualization. Subsequently the difference between misinformation and disinformation is being discussed. Also, the common methods for altering the result and representation of data will be explained. Further it will be shortly discussed what Data really is in an ontological sense. In the end there is a short hypothesis given why Information visualization can have a strong impact on the perception of an individual.
Information and Data
Information Visualization concerns the graphic display of data, and more generally speaking, information. While a clear distinction between data and information is difficult to obtain, it is nonetheless very important. The word ‘information’ comes from the Latin word ‘informatio’, which means concept or outline, and ‘informare’, which means to educate or give form to something. In modern language the communicative meaning of ‘telling something to someone’ is mostly being used. However, the term ‘information’ is commonly used for a lot of different contexts. There is no single, simple explanation for what it is, and the definition is not limited to solely being facts that are provided about something or someone. Saint-Onge (2002) describes information as organized data. However, this narrow definition is heavily disputed. There is a variety of different definitions, depending on the research area in which this question arises. While for example ontology refers to information as the being and entities that exist, Epistemology refers to information as knowledge. It could also be discussed whether there is only one form of information or if there are several.
There is a mathematical approach to the definition of information provided by Claude Shannon: “He defined information as a message sent by a sender to a receiver. […] Shannon gave information a numerical or mathematical value based on probability defined in terms of the concept of information entropy more commonly known as Shannon entropy. Information is defined as the measure of the decrease of uncertainty for a receiver. The amount of Shannon information is inversely proportional to the probability of the occurrence of that information, where the information is coded in some symbolic form as a string of 0 s and 1 s or in terms of some α-numeric code “(Logan, 2012). While Shannon laid the groundwork for the field of information theory, it should be noted that his concepts were not motivated by philosophical questions. Nonetheless, this theory is referred to as a starting point in many philosophical literature for its coherence and clarity. Heuristically speaking, Shannon’s theory regards the information content of a message as high if the event that is messaged is surprising, i.e. has a low probability to occur. In this theory, the information content of a message will be zero if everything transmitted is 100% guaranteed to happen anyway.
Gregory Bateson defines information as “the difference that makes a difference” (Logan, 2012)., which implies that it is the meaning of information that is most important. Another definition that includes the meaning of information is given by Ed Fredkin: “The meaning of information is given by the process that interprets it” (Logan, 2012). This emphasizes that information not only consists of its meaning, but also depends on its context. However, if information is the difference that makes the difference, that would mean if there was no difference, there would be no information. This would mean that chaos or random numbers contain no information because there is no difference in one part of the stream of numbers as opposed to another part of the stream because of a lack of organization. This is opposite to the conclusion of Shannon who claims that a stream of random numbers contains the maximum information. (Compare p.10 Logan, 2012)
Another fundamental factor of information is it´s novelty. The newer a piece of information is, the more informative/relevant it becomes. Old information is no information. This view for example is consistent with Shannon’s theory if one regards old information as widely known and guaranteed to happen (as it already happened), in which case we see that Shannon would assign a message conveying such an information zero informational content.
Data is distinguished from information as the former is not required to be in a form that is accessible. While 100 questionnaires may provide data, they alone are insufficient to provide information, whereas a study based on these questionnaires can provide information. The aggregation and processing of data is necessary to present it as information (Compare Hansson, 2002). While there are multiple different disciplines contributing to the definition of data, all of them focus on different concepts and aspects of said term.
“Information Systems, Science, and Technology explore the formal manipulation of data as abstract symbol sets, relational modelling, and functional data modelling. Semiotics contributes components of a philosophy of signs and their interpretation. Formalizing certain signs into facts is the domain of the Philosophy of Science, discovering the inner nature of facts. The Philosophy of Technology, meanwhile, contributes ethics and value-systems to data, as well as tools to help us probe its fundamental philosophical nature. Information Theory explores the nature of the mechanical transmission of data, imposing physical constraints in our constructed reality. The sciences of Education and Biology both explore the neurological basis of our brains’ understanding of data” (Ballsun-Stanton, Bunker, 2009).
However the further examination of these fields is not the main emphasis of this text.
From Data to Knowledge
The process of gaining knowledge from data involves several steps. As seen above, information has to be created by bringing data in an accessible form. Next, information has to be transmitted. One way to do so is to visualize information, and what this includes will be discussed below. After that, a visualization has to be perceived. Perception is a way of collecting data through our five senses. By organizing, identifying and interpreting this data we generate information. While this is another important step, it is not the focus of this section. Hansson (2002) also remarks on the important observation that information alone is not able to cause knowledge, as this requires the acceptance of information as valid. Without the belief that certain information is true, information cannot and will not cause the creation of knowledge.
Knowledge
Usually, knowledge and information are considered separately. Knowledge is understood to be the stock of facts, theories and rules that have the greatest certainty and are therefore accepted as valid or true. Or to quote Plato: “Knowledge is justified true Belief”. “Justified” in that matter means that you need to know how something comes to be from a scientific point of view. In retrospect, one can claim to “know” future random events (for example the numbers for the lottery) beforehand and say that one would have already known that they happen to be correct ones afterwards. That would be considered as a belief which randomly turned out to be true. But since nobody can justify their opinion about the next random event (e.g. lottery drawing) at a previous point in time, it is not knowledge in the sense of the analytical philosophy, even if the “predicted” numbers are the correct ones again because none can say why these numbers will be correct. (Kelley L. Ross, 2016)
Knowledge can usually be split into “Know how” and “Know that”. “Know how” is implicit, i.e. someone knows how to do it, but cannot say exactly how and why to do something in a certain way (z.B. riding a bicycle). “Know that” refers to propositional knowledge, i.e. knowledge that can be expressed. Information visualization can help to generate propositional knowledge. In terms of analytic philosophy, this kind of knowledge is a true, justified belief. (Ichikawa & Steup, 2017)
People prefer to use their eyes for sensory perception or generally perception and the processing of information and thus generation of their own knowledge or rather beliefs. Whether the perception of an individual is “true” or “false” in the sense of the previously defined manner, does not matter. The perception of an individual can be altered from reality due to many factors such as religious (believes), drug use or some other sensory malfunction. But generally speaking, visual stimuli have the highest information density and therefore create supposedly the best impression and strongest sense of the environment and thus reality. (Wörgötter, o. D.)
Data and information can now be numeric, relational, or textual. Pictorial representations are a method to compress information and to represent/communicate interrelations easily and understandable. Visualization is therefore used to gain knowledge. Thus, Knowledge can be generated by evaluating visualized information. Such a transfer is called knowledge visualization. (Meyer, 2009)
Paradoxically, it is precisely due to the combination between easy understanding and high compression of the information which is why the visual representation of information can easily be used for the purpose of manipulation and influencing. Paradox, because it is very easy to come to a wrong conclusion, although the information is correctly presented, but through the type, connection and way of representation of information it can easily create disinformation or misinformation - for example by using pseudo correlations by purpose.
Because lying with statistics is the easiest way to foster disinformation I’d like to go through the most common ways to do so and explain why that is using the concepts of Ontology, Epistemology, Ethics and Methodology. Keep in mind that every statistic can be visualized and thus create an image in the terms of Visualization. Also, there are a few ways to project Information intentionally or unintentionally wrong via statistics. Through altering the digits in general or graphically by altering the perception of correct numbers, wrong/no context or a combination of each.
Visualization
Visualization can be seen from two points of view, an artistic one and a more technical, analysis oriented one. While the technical form is mostly practiced by people in computer science with no background in art or design, artists and designers often work on visualizations without knowing the technical work being done in computer science (Compare Kosara, 2007). One could assume that visualization is any kind of display that communicates a message. As Fioridi states, “[…] a visualization image is a form of data and conveys information” (Fioridi, 2014). However, according to Robert Kosara (2007), there are several criteria to be required for an entity to be counted as a visualization. First of all, it needs to be based on non-visual data. If the source data is an image and is used as an image in the end, it is not being visualized. Second it has the goal of producing an image. The visual must be the primary means of communicating the data and while other media can be part of the visualization, it must be able to stand on its own. And third, the result must be readable and recognizable. A visualization should be readable by a viewer, even if that requires background knowledge or practice. Also, visualization images must be recognizable as such, and not appear to be something else. (Compare Kosara, 2007).
One could state that visualization is a very important part of information transfer. From childhood on we already learn to interpret signs and images, even before we are able to speak. There even is an old saying that goes: “An image speaks more than thousand words”. And while that may be exaggerated, visualizations do take a big part in presenting data and information. One could go as far and say that even lettering is a form of visualization. A word written in bold print conveys a different message than a normal written one, independent of the actual meaning of the word.
There are a multitude of purposes involved in information visualization, depending on who intends whom to gain which insight. Purposes of graphic displays of information can include for example fostering knowledge, compressing information, presenting complex interconnections, and relations in an easily accessible way, but also influencing or even manipulating the opinions of those who perceive such visualizations.
“However, it is not absolutely factual that all uses of visualization are for gaining a deep understanding, unless the term insight is broadened to encompass all types of thought. Even when insight is the focus of a visualization task, it is rather difficult to know what insight is gained, how much, or how accurate. […] However, insight is a non-trivial concept. It implies ‘accurate and deep intuitive understanding’ […]. While this may be what everyone who creates or uses visualization is inspired to achieve, it is an elusive notion and rather difficult to measure, evaluate, or validate objectively” (Floridi, 2014).
The four Sides Model
The four sides model, created by German psychologist Friedemann Schulz von Thun, is a model of communication, understanding and misunderstanding. He talks about a sender and a receiver, about the perception of a message and how analysis can lead to misunderstanding. The model says that a message can be sent and understood in four different ways. The sender is the person sending a message by saying or writing something. The receiver is the one receiving the message by listening or reading it. The message is what's being said or written. Now there are four different facets of the sender’s intention and the reader's perception. First there is the factual information. It contains objective information like observed data and indisputable facts. Second is the appeal. It contains commands, advice, or instructions that the sender wants to give. Third is the Relationship. It contains information on the relationship between sender and receiver, what they think of each other and how they get along. Fourth is self-revelation. It contains implicit information, intended or unintended, about the motives of the sender. Now when the sender transmits a message, it is sent with the intention of one of the four sides. When the receiver gets the message, it is received/interpreted through one of the four sides as well. The communication between the two is successful if they both send and interpret the message through the same side.
As information visualization is a nonverbal way of communicating, this model can be transferred to the topic. Let information visualization be the message, let the sender be the one creating the visualization and the receiver be the one looking at it and interpreting it. Again, there are four sides to analyze.
Beginning with the factual information, we have the pure information content of the visualization displayed. Here, one can ask, whether the pure information content is factually correct, which will later be discussed regarding misinformation and disinformation. One can also ask whether a visualization provides a sufficiently complete picture of some information. Then we have the appeal. There are visualizations that are explicitly targeted to show a particular fact. An image/graphic can be specifically created in a way that directs the viewers’ attention towards a certain direction. Take for example a newspaper article about the covid-19 pandemic with a picture of a vaccine under it, an image that creates hope for most people. Now imagine the same article, but with a picture of a sick person on an intensive care unit, an image that conveys the misery of the pandemic. The same article with different visualizations can invoke positive or negative associations. Therefore, it is apparent that the appeal of an information visualization is not always for the receiver to gain knowledge. Rather, its appeal can be misinformation or even manipulation, in the sense that the intended reaction of the receiver.
Third, we have the side of the relationship. The way that the content of a visualization is created/displayed acts in accordance with the target audience. Data can be shown in its raw form or supplemented with additional annotations. In fact, it was stated above that some view this processing step as integral to create information from raw data. A teacher presenting their students a complex data visualization without additional commentary and subsidiary information demonstrates a higher level of trust in their student’s ability to correctly interpret and analyze such a visualization than a teacher that guides their students through every detail by providing a heavily annotated form of visualization of the same data. The important takeaway is that an information visualization is never independent of the relationship between the sender providing such a visualization and the receiver who sees it. Lastly, there is the side of self-revelation. The sender reveals information about himself by depicting something in a certain way. Take for example a political cartoon, in which the artist makes fun of a certain party. The message of the cartoon will reveal the artists opinion about said party. The choice of which information to visualize can indicate the intentions of and biases held by the sender.
Therefore, the implications of the 4 sides model transfer to the case of information visualization and highlight various integral aspects that are important in order to assure successful information transfer. For example, any form of information visualization is firmly connected to both the sender and the receiver. A visualization suitable for professionals might easily become deceptive if presented to an audience without the necessary background knowledge.
Misinformation and Disinformation
According to the dictionary, misinformation is “false information that is spread, regardless of intent to mislead” (dictionary.com/ accessed 02.06.2021). This means that if an individual shares information that is wrong but said individual doesn't know that it's wrong, they are spreading misinformation. This occurs regularly in our everyday life. As we currently live in an information era, misinformation can easily be spread through the media, especially social media, on platforms like Facebook or Instagram.
On the other hand, we have disinformation. It is defined as “deliberately misleading or biased information; manipulated narrative or facts; propaganda. So, disinformation is knowingly spreading misinformation” (dictionary.com/ accessed 02.06.2021). This occurs for example on a political level, where deliberately spread, disinformation can lead to tactical political demolition. Deliberately spread disinformation is also used in propaganda, mostly practiced in modern dictatorships, to present something in a good or a bad light. Bernd Carsten Stahl defines “misinformation as accidental falsehood and disinformation as deliberate falsehood. […] one can say that for a critical researcher misinformation are such claims that inadvertently lead to alienation whereas disinformation are claims which the originator knows to be alienating but nevertheless proposes” (Stahl, 2006). This begs the question if deliberately held back information does also count as disinformation. Withheld evidence in a court case that may lead to an unjust conviction provides a compelling argument in favor of that view. To conclude one could state that “misinformation is not problematic. It is simply information that is contentious and that therefore will be analyzed in a discourse. The person claiming truth will have to explain the reasons for the claim and will have to answer critique. All of this can be done within the framework of communicative action where people recognize each other as dignified beings and are willing to take each other seriously. Disinformation is more problematic. Since it is information that deliberately alienates or disempowers people, the speaker shows a disregard for the other who is disempowered” (Stahl, 2006).
One difficulty that arises in the specific case of information visualization is that the visualization if often spread beyond the control of the sender. The ‘framework of communicative action’ referred to above can be heavily impaired, which prevents an effective framework to resolve issues of misinformation. Another aspect important to information visualization is that it becomes increasingly difficult to draw a line between misinformation and disinformation. A visualization intended to mislead (disinformation) might be further spread by those which are unaware of the deception (misinformation). The same visualization can therefore be both misinformation and disinformation. One possible conclusion among many to draw here is the one provided by the four sides model, namely that information visualization (as a message) is never disconnected from the relationship between sender and receiver.
Examples on how misleading can occur
Hidden parameters
One of the most commonly known examples of a spurious correlation (without bad intent of course) is the connection between the birth rate and the number of storks in Germany - Shown in the graph below.
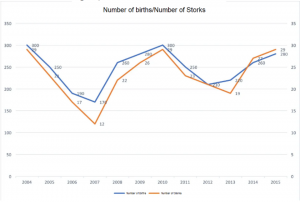
There is obviously no real connection between both numbers but at first just by looking at both graphs it can create a strong impression that there is one because both graphs climb and fall at around the same time and rate and the human brain is really effective in finding alike patterns and shapes. In this first example with the storks it happens to be that storks usually are not living in urban areas and the birth rates in urban areas are lower. So the real parameter behind this correlation is the urbanization of the area. (Keller, 2013)
If you’d pick a less obvious connection between two readings, for example the number of tomatoes eaten and obesity in the USA, you’d probably end up finding a similar pattern. If one doesn't have much knowledge in statistics, one may confound correlations with causality and may come to the conclusion that tomatoes are the reason for obesity in the USA. This example tells at first that tomatoes are causing obesity. But because Tomatoes are commonly used in salads, dips or dishes in general, it’s obvious that the real factor is down to the overall food consumption.
Another example would be the comparison of the age of death between teachers and professional football players. Teachers are dying at a much higher age compared to football players. You can think of a multitude of reasons for that. It could be a very high stress level for the body that causes such a low age of death, maybe headers are the reason. In all three examples there is a hidden parameter causing these correlations. This third example compares two groups that can’t be compared this way because the average age is much different. Whilst the profession of teaching exists for hundreds of years, real professional football players do exist for around 60 years. Also, there are many more teachers than football players. If a football player dies, he is on average much younger, because the profession doesn’t exist for that long and there are much fewer in total. So a death in that case carries much more weight. (Lügen mit Statistiken, o. D.)
Feint with graphs
Feinting with graphs involves altering scales or highlighting important points in graphs in excessive way. Mostly it happens with faulty scales between two objects of the same kind, or altering intervals f.e. logarithmic intervals, or if a graph doesn’t start at zero. (Lügen mit Statistiken, o. D.)
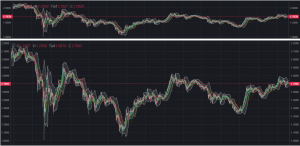
In the example above, the first chart appears to be not as volatile as the second chart. But both charts show the same asset with different resolution of the value (vertical axis) in relation to time (horizontal axis).
Apparent accuracy
An apparent accuracy describes a resolution for results that can’t be provided by the underlying data and measurements. There are three basic reasons for the apparent accuracy. Firstly, Significant digits are not taken into account. A computer got input without any restraints and the output were taken without taking in accuracy of the input data. This is closely related to the first reason. Lastly, results with apparent accuracy seem for an individual more reliable than the correct counterpart. We as humans tend to think that these digits are more trustworthy because most of us think that more digits mean more care while measuring values. This can be used on purpose.
“[...] an economic report states that 1,450,000,000 overtime hours were done in Germany each year.[...] for 40,000,000 employees this would mean that every employee did exactly 36.25 hours of overtime per year.[...]” In that example you can’t tell how much each employee really did, but it seems that you know. In that case a range has to be given. F.e. one to two-billion hours overtime and each employee worked between 30-50 hours longer each year. “Herodotus wrote after the Persian War that the enemy army consisted of exactly 5.283.220 men.” In this case Herodotus can’t be able to tell the exact number. The actual number was around 15,000 soldiers - In this case he chose such a number because of the general perception of people that such numbers are more precise, because they are supposedly more carefully counted. He knowingly lied, to appear more successful than he really was. (Lügen mit Statistiken, o. D.)
Disingenuous Samples, ambiguous Questioning, concealed realities
An important method for gathering information in science are polls. The Information polls are usually opinions, knowledge or behavior patterns of people. The significance of the data comes down to how the data was collected, how widely the sample size was chosen, how questions were asked etc. The following common errors are usually rooted in real numbers and thus can be communicated in a correct way but were collected with faulty or intransparent methods. (Lügen mit Statistiken, o. D.)
A Disingenuous sample comes to be if some mandatory rule of data acquisition was knowingly or unknowingly broken. An ambiguous question sometimes comes in a subtle way, when someone has a certain bias for example. But if someone wants to prove their own point, this is a method to manipulate participants of surveys into answering in a certain way. In all cases the result of a survey provides a distorted image of reality. (Lügen mit Statistiken, o. D.)
A Disingenuous sample for example accrues if you asked environmentalists at a demonstration if they like sport cars or not, and conclude based on the answerers that people don’t like sport cars. If you did the same at the automotive international motor show you’d most certainly get the opposite results. If you’d ask the same people at the automotive international motor show if they are pro ecology and against combustion engines - you’d ask an ambiguous question. Most of them would agree because they don’t want to appear reckless about the environment.
Concealed realities in terms of statistics accrue when the real basis of an evaluation is unknown. This can happen if a basis contains more information than one can guess with common sense. (Lügen mit Statistiken, o. D.)
If you pick the number of unemployed people in a country you need to know who exactly counts as unemployed. You are officially counted as unemployed in Germany if you are willing to work more than 18 hours a week, and are available for the workforce. This means that anybody that wants to work but can’t meet the 18 hours mark per week and is unemployed, like a student for example, doesn’t count as unemployed. That means that if no student had a job and can’t work at least 18 hours a week, but everyone could work 15 hours, none of them would count as unemployed, leaving millions of people unconsidered on the basis of the statistic.
Bigoted percentages, deceit with averages, manipulated basis
The following are more related to altering the digits in a certain way. You deal with a manipulated basis when someone takes a subset of a database and refers to it as the base. If this subset contains the factors someone wants to compare but are more closely related to the real base, meaning it makes more sense to compare it with the real base, it can significantly alter the results (especially if relative numbers are chosen for representation). (Lügen mit Statistiken, o. D.)
If 8 of 10 deaths of cyclists occur despite wearing a helmet, you could conclude that wearing a helmet is dangerous. In this case you take the subset of killed cyclists and compare the two outcomes dead or alive. However, if 9500 cyclists are wearing helmets and 500 don’t and assuming that the possibility of an accident is equal in both cases, you’d find that the chance of dying is actually 5 times higher without wearing a helmet.
Another method to blur reality with relative numbers is comparing two bases of the same unit but much different starting points. These bigoted percentages can tell the observer a tremendous relative increase or decrease and hide the absolute numbers behind it, which are needed to tell the whole story. (Lügen mit Statistiken, o. D.)
Imagine two companies A and B in the same country. Both of them operate with a capital of €100,000,000. Both are industrial companies that produce gearing mechanisms. Company A generated a profit of €1,000 in the year 2019 and a profit of €3,000 in the year 2020. Company B generated a profit of €1,500,000 in the year 2019 and €1,800,000 in 2020. Because the walls for the CEO of company A are closing in, he states that he managed to increase profit within a year by 300% and states that company B were only able to increase profit by 20%.
The same CEO also claims that orders also increased by 100% on average over the last year. In 2019 company A got 10 orders on average per month from. In 2020, they were able to raise the number to 20. This is true, but he just got 60 extra orders in March and April because a car company desperately needed gears for the production of convertibles that summer and company A was dropped shortly after they delivered due to major quality issues. In this case, deceit with averages was done by using an outlier to boost the average. An alternative to averages are medians. In this case the median would be still 10.
Rationale
After describing how someone can create a blurred image of reality with Bigoted percentages etc., there has to be a reason that can be explained with the concepts of ontology and/or epistemology why a receiver might believe in them. Topics of ontology are the question of existence, the problem of totality, but also the question of correlation and context. (Wikipedia : Ontologie, 2021)
First, Ontology distinguishes abstract objects and concrete objects. This by most philosophers agreed to be the most generalized categorization of being. Concrete objects are in that matter physical objects like a stone for example. Numbers and digits are abstract objects. (Rosen, 2017)
Therefore, statistics are abstract objects because they are based on numbers. There is also an otologic dependency and causal dependency. Otologic dependence means that an entity is dependent on another entity and can’t exist on its own. Causal dependency means that the effect of an entity exists because of another entity. (Esfeld, 2002)
Another important role in discussing this topic are relations and relational properties. Relations are n-ary. N-ary means that there are n objects or entities that can be related to one another. If x and y are related, it’s binary because there are two entities (x and y) that are related. A relational property holds the information why x is related to y or vice versa. A relational property can also be n-ary. Relations can be symmetric, asymmetric or non-symmetric. “A binary relation R is symmetric if whenever x bears R to y, y bears R to x. By contrast, R is non-symmetric if R fails to be symmetric. Asymmetric relations are a special case of non-symmetric ones: R is asymmetric if whenever x bears R to y then y does not bear R to x. So whilst all asymmetric relations are non-symmetric, not all non-symmetric relations are asymmetric.” (MacBride, 2020)
To sum it up, a statistic is an abstract entity that relates two sets of data in a symmetric or asymmetric oncologic dependent way without those sets of data necessarily being causal to each other. This entity can now be expressed as some kind of image.
In philosophy, it is possible to differentiate between actuality and reality. In this case, reality regards physical objects that can have a real effect in the real world. They are both in actuality and reality. Abstract objects like numbers are part of reality but not of actuality. But in Platonism they can exist without someone thinking about them. (Balaguer, 2016)
“Causation appears to be multigrade because a certain number of events may be required to bring about an effect on one occasion, and a different number of events may be required to bring about an effect on another.” (MacBride, 2020)
Therefore, Causation is a symmetric or asymmetric relation between at least two physical objects that affect each other and exists in actuality and reality. The relation and result between can always be expressed numerically. This comes naturally but is not always obvious. The amount of beer some people drink in one hour is causal and correlates to how drunk the person is. The relation can be measured in the amount of alcohol and the result can be observed, and the typical neurological deficits can be put into relation to the amount of blood alcohol (i.e. consumed beer). There is a symmetric relation (amount of blood alcohol and consumed beer), or asymmetric relation (neurological state and blood alcohol). The neurological state could be measured by using brain activity, for example.
Hypothesis
People naturally search for connections and context, the brain is great in creating patterns and connections without those even existing. Thus, we tend to recognize and rationalize the images and connections between data we see according to relations, despite the fact that relations are not always causation. This also comes down to our own sense of reality that can lead us into selectively ignoring the truth, just to underline our own beliefs. (Wörgötter, o. D.)
The goal of Information visualization is to provide the observer with information. The observer processes the information. The processing depends on the individual perception of reality/world view and ability to recognize patterns. (Philosophie Magazin : Wahrnehmung, o. D.) The individual may or may not be more likely to overlook the previously described traps that can intentionally or unintentionally be a part of statistics and the visualization of Information/Knowledge. However, if people are aware of these tricks/flaws they usually spot them right away.
References
- Balaguer, M. B. (2016). Stanford Encyclopedia of Philosophy : Platonism in Metaphysics. Stanford Encyclopedia of Philosophy. https://plato.stanford.edu/entries/platonism/
- Ballsun-Stanton, B. & Bunker, D. (2009). Philosophy of Data (PoD) and it´s Importance to the Discipline of Information Sciences. Conference: Proceedings of the 15th Americas Conference on Information Systems, AMCIS 2009, San Francisco, California, USA, August 6-9
- Carsten Stahl, B. (2006). On the Difference or Equality of Information, Misinformation, and Disinformation: A Critical Research Perspective. Informing Science: The International Journal of an Emerging Transdiscipline, 9, 083–096. https://doi.org/10.28945/473
- Dictionary.com. (2021, 19. Januar). “Misinformation” vs. “Disinformation”: Get Informed On The Difference. https://www.dictionary.com/e/misinformation-vs-disinformation-get-informed-on-the-difference/
- Esfeld, M. E. (2002). Michael Esfeld über Ontologische Abhängigkeit – Lexikon der Argumente. Philosophie Lexikon der Argumente. https://www.philosophie-wissenschaft-kontroversen.de/details.php?id=252770&a=t&autor=Esfeld&vorname=Michael&thema=Ontologische%20Abh%C3%A4ngigkeit
- Floridi, L. & Illari, P. (2016). The Philosophy of Information Quality (Synthese Library, Band 358) (Softcover reprint of the original 1st ed. 2014 Aufl.). Springer. http://ndl.ethernet.edu.et/bitstream/123456789/9511/1/109.pdf
- Hansson, S.O. (2002). Les incertitudes de la société du savoir. Revue international des
- sciences sociales, 1 Nr. 171, pages 43-51
- Ichikawa, J. J. I. & Steup, M. S. (2017). Stanford Encyclopedia of Philosophy : The Analysis of Knowledge. Stanford Encyclopedia of Philosophy. https://plato.stanford.edu/entries/knowledge-analysis/
- Kelley L. Ross, K. L. R. (2016). Friesian : Knowledge. friesian.com. https://www.friesian.com/knowledg.htm
- Keller, D. K. (2013, 21. Mai). Statistik und Beratung : Von Störchen und Babys: Die partielle Korrelation. Statistik und Beratung. https://statistik-und-beratung.de/2013/05/von-storchen-und-babys-die-partielle-korrelation/#:~:text=In%20einer%20Studie%20wurde%20f%C3%BCr,mehr%20Babys%20gibt%20es%20dort.
- Kosara, R. (2007). Visualization Criticism – The Missing Link Between Information Visualization and Art. The University of North Carolina at Charlotte
- https://kosara.net/papers/2007/Kosara-IV-2007.pdf
- Logan, R. K. (2012). What Is Information?: Why Is It Relativistic and What Is Its
- Relationship to Materiality, Meaning and Organization. Information, 3(1), 68–91. https://doi.org/10.3390/info3010068
- MacBride, F. M. (2020). Stanford Encyclopedia of Philosophy : Relations. Stanford Encyclopedia of Philosophy. https://plato.stanford.edu/entries/relations/#ElimExteRelaBradRegr
- Meyer, R. M. (2009). Ludwig Maximilians Universität : Knowledge Visualization. Ludwig Maximilians Universität. https://www.medien.ifi.lmu.de/lehre/ws0809/hs/docs/meyer.pdf
- Lügen mit Statistiken. (o. D.). Statista. Abgerufen am 24. Mai 2021, von https://de.statista.com/statistik/lexikon/definition/8/luegen_mit_statistiken/
- Wikipedia : Ontologie. (2021, 31. Mai). Wikipedia. https://de.wikipedia.org/wiki/Ontologie
- Rosen, G. R. (2017). Stanford Encyclopedia of Philosophy : Abstract Objects. Stanford Encyclopedia of Philosophy. https://plato.stanford.edu/entries/abstract-objects/#:~:text=An%20object%20is%20abstract%20(if,distinct%20from%20its%20concrete%20instances).
- Saint-Onge, H. (2002). Linking Knowledge to Strategy. Presentation at the Strategic Planning for KM Conference, Toronto, May 28-29
- Wörgötter, M. W. (o. D.). Hochschule Augsburg : Typografie Beurteilung Selektive Wahrnehmung. Hochschule Augsburg. Abgerufen am 28. Mai 2021, von https://www.hs-augsburg.de/~brownfox/brownfox_dokumente/Typo_1/Typografie_Beurteilung_Wahrnehmung.pdf
- Philosophie Magazin : Wahrnehmung. (o. D.). Philosophie Magazin. Abgerufen am 31. Mai 2021, von https://www.philomag.de/lexikon/wahrnehmung